Recent publication of the Autofair project proposes a fairness-aware mechanism for forecasting of time-series. When applied to estimating risk of reoffending, this has produced the present-best results on a dataset known as COMPAS, from the system utilized in judicial systems of California, New York, and Wisconsin.
In machine learning, training data often capture the behaviour of multiple subgroups of some underlying human population. This behaviour can often be modelled as observations of an unknown dynamical system with an unobserved state. When the training data for the subgroups are not controlled carefully, however, under-representation bias arises.
To counter under-representation bias, the paper introduces two natural notions of fairness in time-series forecasting problems: subgroup fairness and instantaneous fairness. These notions extend predictive parity to the learning of dynamical systems. The paper also show globally convergent methods for the fairness-constrained learning problems using hierarchies of convexifications of non-commutative polynomial optimisation problems. It also shows that by exploiting sparsity in the convexifications, one can reduce the run time of our methods considerably. The empirical results on a biased data set motivated by insurance applications and the well-known COMPAS data set demonstrate the statistical performance and a number of criteria for the evaluation of fairness, improving upon the state of the art as implemented in the AI Fairness 360 toolkit.
See the publication: Zhou Q, Mareček J, Shorten R (2023): Fairness in Forecasting of Observations of Linear Dynamical Systems. Journal of AI Research, Vol. 76.
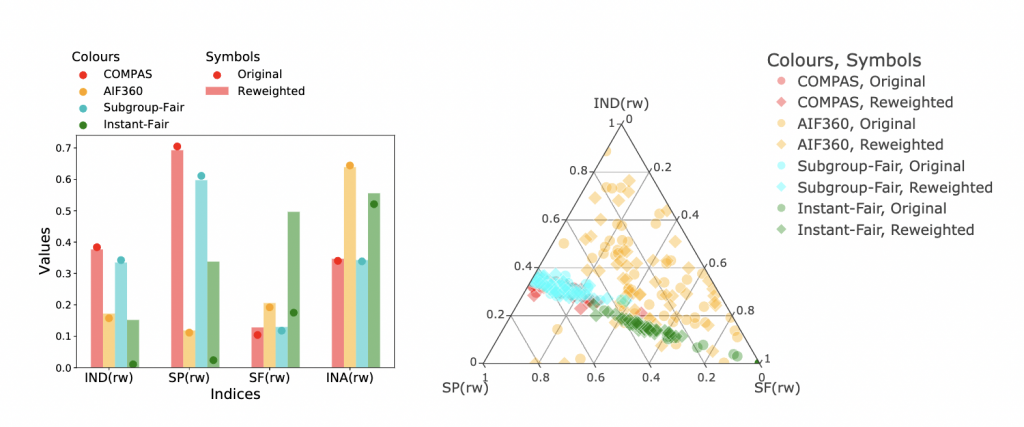